Googleの機械学習専用カスタムチップはGPU/CPUマシンの15〜30倍速い...同社ベンチマークを発表
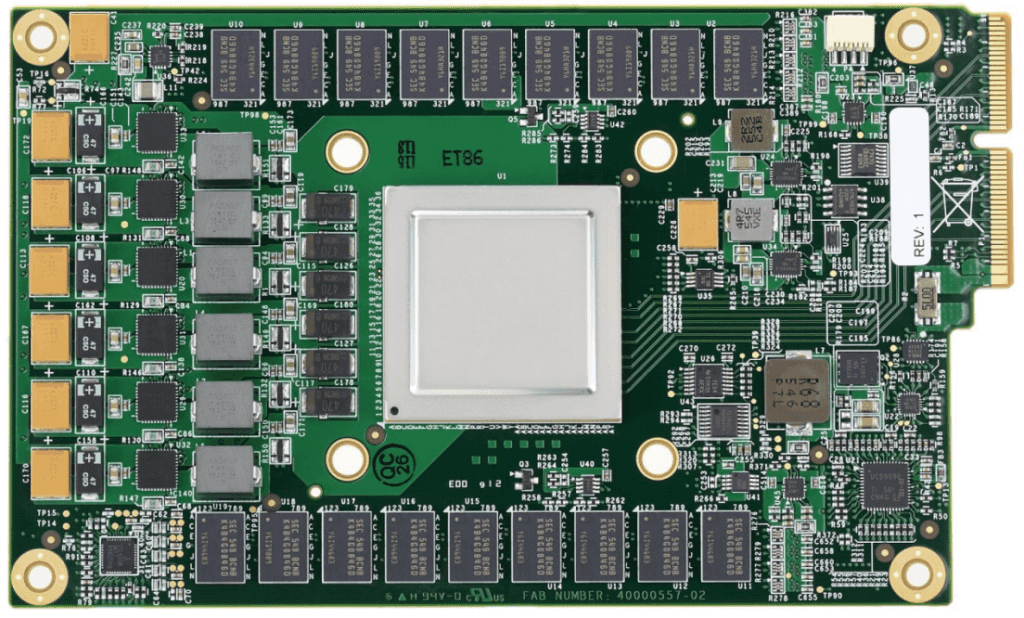
【抄訳】
Googleが同社の機械学習アルゴリズムを高速に実行するカスタムチップを独自に開発したことは、前から知られていた。その Tensor Processing Units(TPU)と呼ばれるチップが初めて公開されたのは、2016年の同社のI/Oデベロッパーカンファレンスだったが、詳しい情報は乏しくて、ただ、同社自身の機械学習フレームワークTensorFlowに向けて最適化されている、という話だけだった。そして今日(米国時間4/5)初めて、同社はこのプロジェクトの詳細とベンチマークを共有した。
Googleが同社の機械学習アルゴリズムを高速に実行するカスタムチップを独自に開発したことは、前から知られていた。その Tensor Processing Units(TPU)と呼ばれるチップが初めて公開されたのは、2016年の同社のI/Oデベロッパーカンファレンスだったが、詳しい情報は乏しくて、ただ、同社自身の機械学習フレームワークTensorFlowに向けて最適化されている、という話だけだった。そして今日(米国時間4/5)初めて、同社はこのプロジェクトの詳細とベンチマークを共有した。
チップの設計をやってる人なら、Googleのペーパーを読んで、TPUの動作に関するややこしいすばらしい詳細情報を理解できるだろう。でもここで主に取り上げたいのは、Google自身のベンチマークの結果だ(客観的な第三者の評価ではない)。それによるとTPUは、Googleの通常の機械学習のワークロードを、標準のGPU/CPU機(IntelのHaswellプロセッサーとNvidia K80 GPUs)より平均で15〜30倍速く実行できた。また、データセンターでは電力消費が重要だが、TPUのTeraOps/Wattは30〜80倍高い。将来は高速メモリの使用により、これよりもさらに高くなるという。
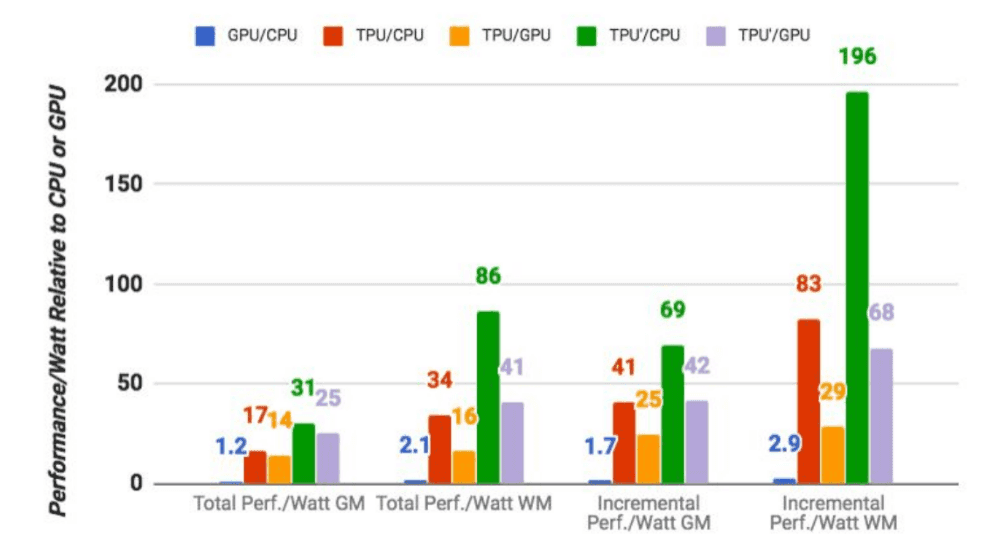
なお、これは実際に使われている機械学習モデルを使った場合の数字であり、モデルの作成は関わっていない。
Googleによると、一般的にこの種のチップはconvolutional neural networks(畳み込みニューラルネットワーク、画像認識などによく使われる)向けに最適化されることが多いが、Googleのデータセンターのワークロードではこの種のネットワークは全体の約5%にすぎず、大多数はmulti-layer perceptrons(多層パーセプトロン)のアプリケーションだ、という。
【中略】
Googleには、TPUを自分のクラウドの外で可利用にする気はないようだが、しかし同社は、これを勉強した誰かが将来、彼らが作る後継機により、“バーの高さをさらに上げる”ことを期待する、と言っている。
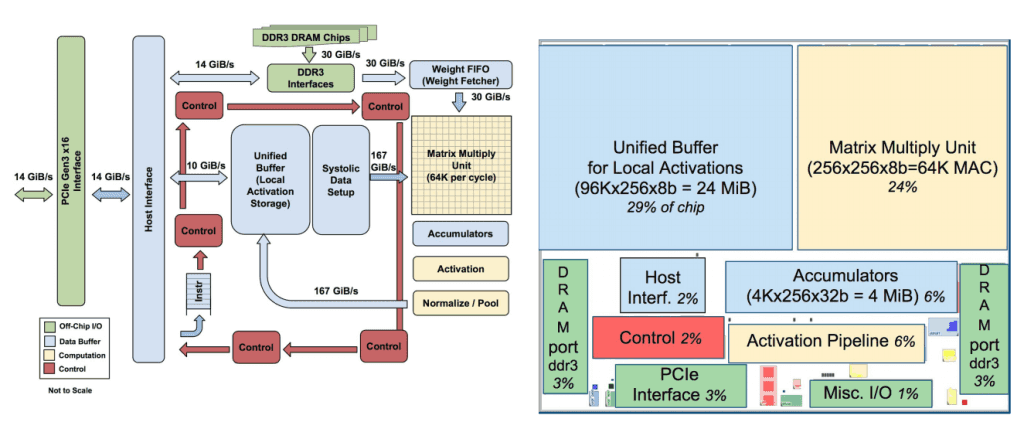
[原文へ]
(翻訳:iwatani(a.k.a. hiwa))
(翻訳:iwatani(a.k.a. hiwa))
Google's dedicated machine learning chips are 15 to 30 times faster than GPU/CPU machines... Announcing its benchmarks. Google's dedicated machine learning chips are 15 to 30 times faster than GPU/CPU machines... Announcing its benchmarks.
Google's dedicated machine learning chip is 15 to 30 times faster than GPU / CPU machine ... announces its benchmark
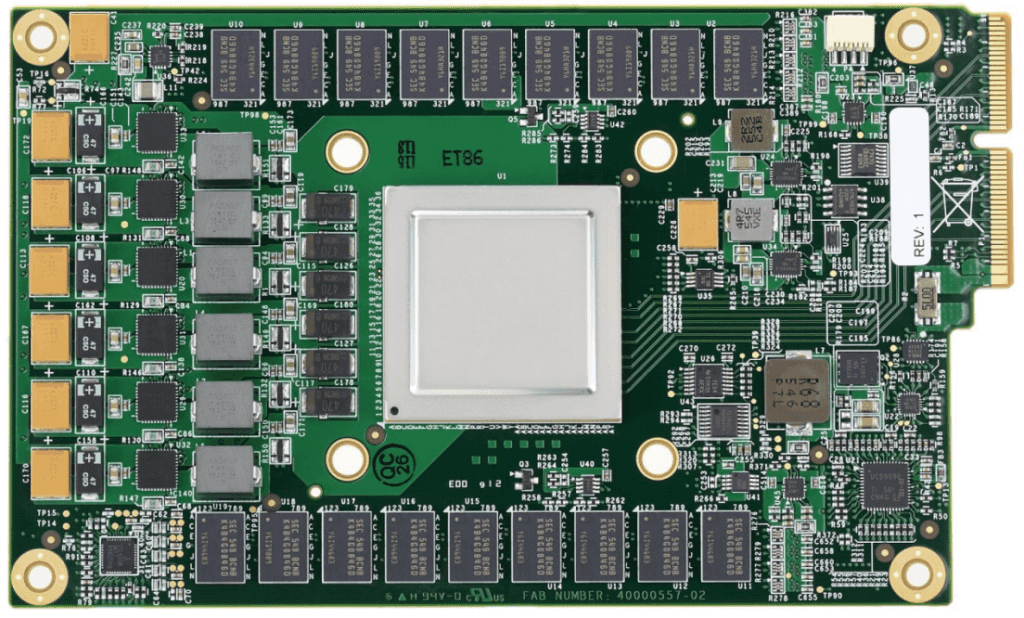
[Abridged]
that Google has independently developed a custom chip to run the company's machine learning algorithms at high speed, has been known from before. The chip called Tensor Processing Units (TPU) was first unveiled at the company's I/O developer conference in 2016, but the details are scarce, but only for the company's own machine learning framework TensorFlow. It was just that it was optimized. And for the first time today, the company shared details and benchmarks for the project.
that Google has independently developed a custom chip to run the company's machine learning algorithms at high speed, has been known from before. The chip called Tensor Processing Units (TPU) was first unveiled at the company's I/O developer conference in 2016, but the details are scarce, but only for the company's own machine learning framework TensorFlow. It was just that it was optimized. And for the first time today, the company shared details and benchmarks for the project.
If you're a chip designer, you can read Google's papers for some great, confusing details about how a TPU works. But the main focus here is on Google's own benchmark results (not an objective third-party rating). The TPU was able to run Google's normal machine learning workloads on average 15-30 times faster than standard GPU/CPU machines (Intel Haswell processors and Nvidia K80 GPUs). In addition, power consumption is important in data centers, but TPU's TeraOps/Watt is 30 to 80 times higher. It will be even higher in the future due to the use of high-speed memory.
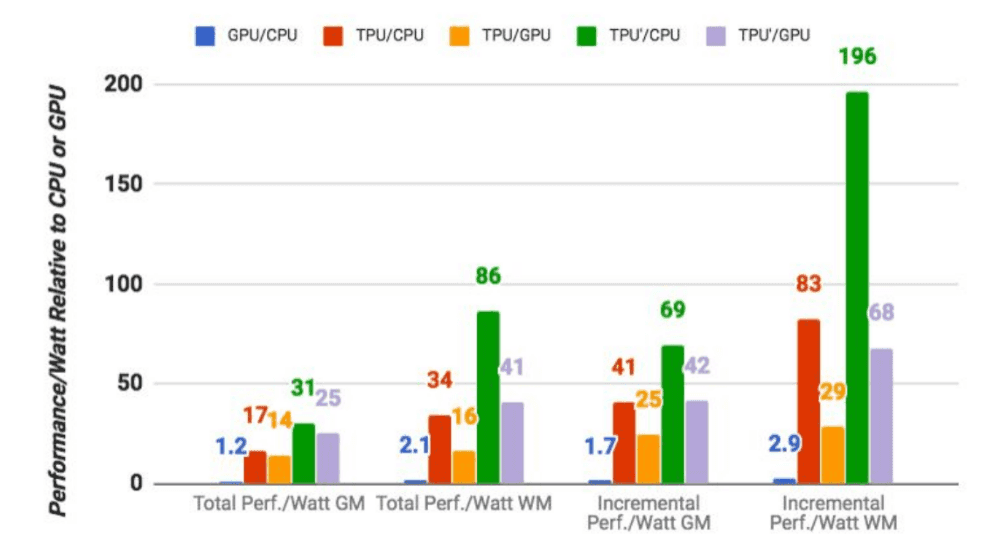
Note that this is the number when using a machine learning model that is actually used, and the creation of the model is not involved.
According to Google, this type of chip is often optimized for convolutional neural networks, which is often used for convolutional neural networks and image recognition. Networks account for only about 5% of the total, and the majority are applications for multi-layer perceptrons.
[Omitted]
Google doesn't seem to be willing to make the TPU available outside of their cloud, but the company "raises the bar height further" by someone who has learned this in the future, with a successor they make. I expect that.
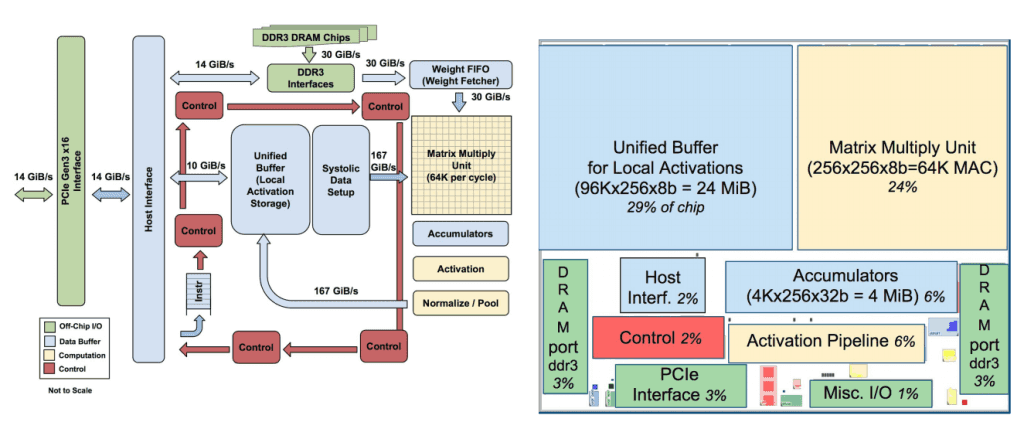
[To original text]
(Translation: iwatani(aka hiwa))
(Translation: iwatani(aka hiwa))
0 コメント:
コメントを投稿