Reprinted for study.
https://note.mu/datastrategy/n/nbbf724464d77
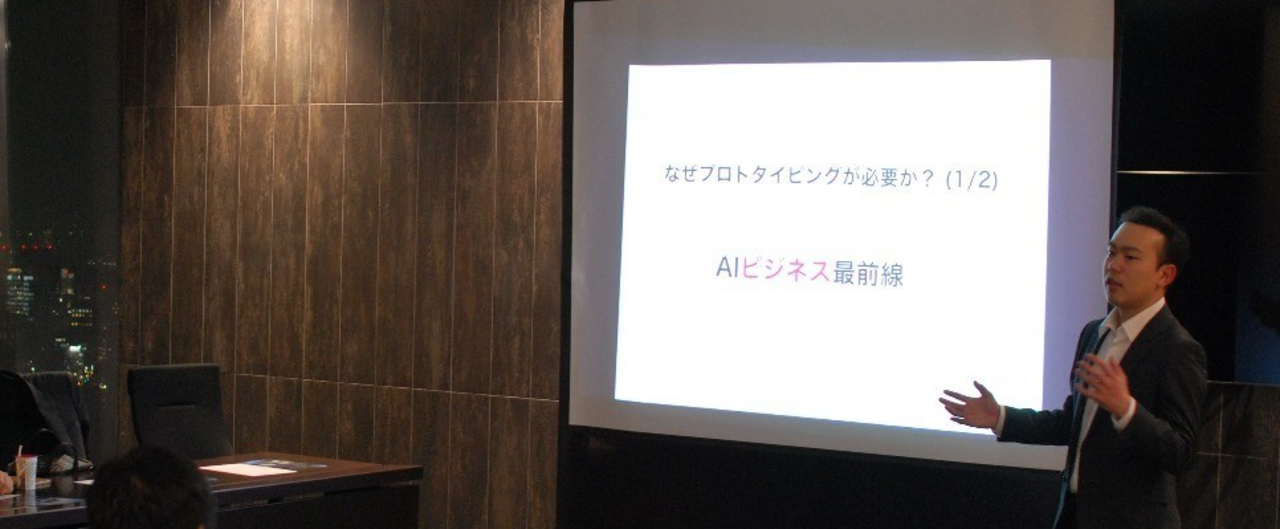
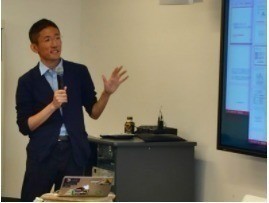
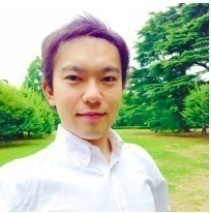
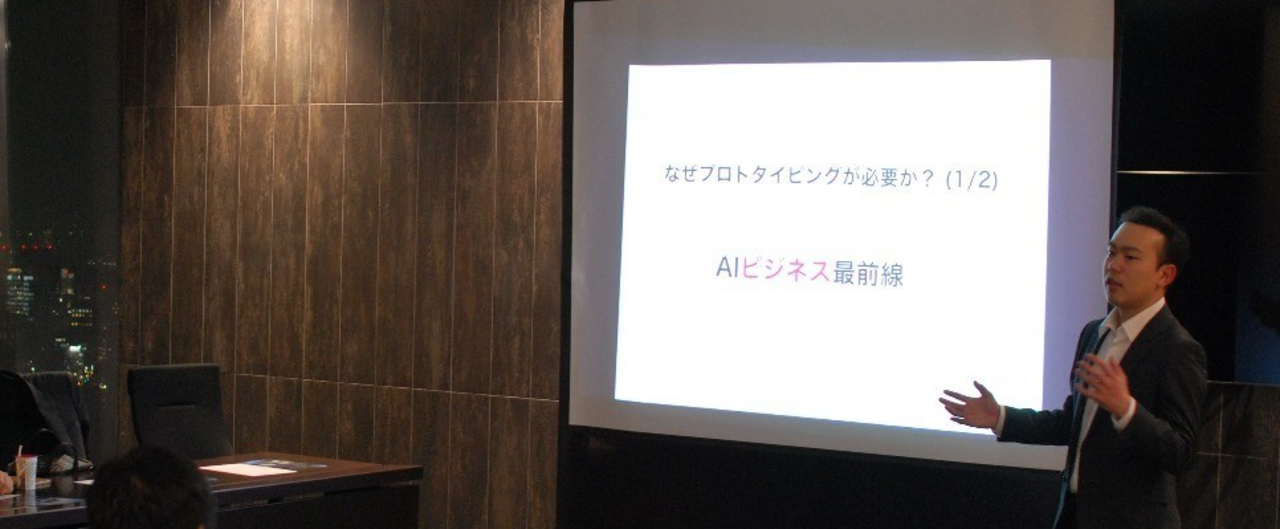
2017 was a year in which various tools related to AI (Artificial Intelligence) were announced and the need to incorporate AI into business was strongly recognized. It was also the year that many companies began to consider how to utilize AI for their business. I think that in 2018, more companies will move to the execution stage, and there will be more scenes where you can feel the impact of AI on your business.
In this article, DataStrategy Co., Ltd., which handles AI and data introduction strategies, and AnyTech Co., Ltd., which provides AI prototyping services, gain insight into the tendency of projects that do not work well among many projects that they have worked on, and hints for success. I analyzed it in a dialogue format . In particular, it focuses on "deployment failures."
The article is divided into two parts, the first half and the second half. The first half is based on common causes of failure, and the second half is based on the experience that "this way it will be better". I would like to introduce it to people who have introduced AI but are worried that it will not work well.
Motohiko Takeda
DataStrategy Co., Ltd. President
https://datastrategy.jp
Graduated from the University of Tokyo (Master of Economics). Worked at Mitsubishi Research Institute, Inc., NPO group, and freelance before assuming current position. So far, he has experience in data analysis support, technology consulting, marketing strategy formulation support at major mobile carriers, commercial banks, and technology venture companies. Engaged in machine learning, artificial intelligence model development, service prototype development, etc.
DataStrategy Co., Ltd. President
https://datastrategy.jp
Graduated from the University of Tokyo (Master of Economics). Worked at Mitsubishi Research Institute, Inc., NPO group, and freelance before assuming current position. So far, he has experience in data analysis support, technology consulting, marketing strategy formulation support at major mobile carriers, commercial banks, and technology venture companies. Engaged in machine learning, artificial intelligence model development, service prototype development, etc.
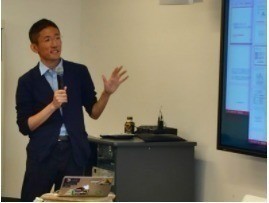
Yoshinori Shimamoto
AnyTech Co., Ltd. Representative Director
https://www.anytech.io/
Born July 1, 1986. Completed graduate school at Waseda University (Master of Engineering). Engaged in development at several companies including the Big Data Department of Recruit Technologies Co., Ltd. App Award at the Watson Development Contest hosted by IBM. Established AnyTech Co., Ltd. and provided AI prototyping service "AnyTech". It has been adopted by Japan's first AI venture support program "AI.Accelerator" and accelerator "TECH LAB PAAK" operated by Recruit.
AnyTech Co., Ltd. Representative Director
https://www.anytech.io/
Born July 1, 1986. Completed graduate school at Waseda University (Master of Engineering). Engaged in development at several companies including the Big Data Department of Recruit Technologies Co., Ltd. App Award at the Watson Development Contest hosted by IBM. Established AnyTech Co., Ltd. and provided AI prototyping service "AnyTech". It has been adopted by Japan's first AI venture support program "AI.Accelerator" and accelerator "TECH LAB PAAK" operated by Recruit.
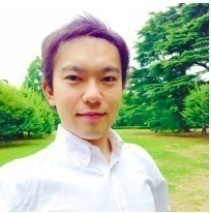
The reason why it doesn't work is because of the idea of the development process and the development tools
--There is a story from a company that introduced AI that it was "more difficult than I expected" or "I couldn't do what I had originally planned." Please tell us the story of “There was such a case”.
Shimamoto : There are two reasons why it doesn't work. The first is that the development period will be extended, and the second is the selection of development tools.
The first is to lengthen the development period from the beginning, including verification of whether data is sufficient, etc., and the subsequent project progress does not go well, resulting in an accurate engine. There is a situation where does not go up.
--Are the development periods not too short, but too long?
Shimamoto : That's right. Perhaps there are cases where the design was based on the idea of large-scale business system development up to now. The AI development process differs greatly from the conventional large-scale business system development process . In the case of AI development, I find it difficult to completely define the requirements before systemizing the actual data in the first stage. There are cases where even small changes and errors cannot be tolerated when it comes to business systems, and I think that there are times when system requirements are rigorously determined, but that may be a major change.
--In business system development projects, it is routine to put things such as intermediate artifacts and milestones in between, but is it also applicable to the introduction of AI?
Shimamoto : That is also different. In system development, I think we can put a milestone (a milestone to be set in the middle for progress management) called "By when this function will be used". However, in the case of AI development, the flow is such as tuning once it has been shaped . You can put milestones, but your thinking will change.
There are many cases in which large companies do not have the proper development period. Well-known companies have many years of relationships with their customers, and often work with well-known companies. This often happens when you work with a development company that specializes in business systems.
Regarding the second development tool selection, some development companies have a constraint that they only use tools and engines that they already have. There are also cases where the development company has a partner agreement with somewhere. There are cases where you cannot optimize for what you are actually trying to create, with the constraint that you have to use that tool .
Since we know the strengths and weaknesses of existing tools, we may start with the consultation and proceed with the subsequent projects. The use of various tools may be reflected in the appeal of AnyTech.
There may be a cause for the selection of the introduction target or the expected value control
-- So that's it. How about Mr. Takeda?
Takeda : I think there are three major cases that do not work.
The first is "I tried to make it, but I introduced it, but the accuracy does not improve." The reason is on a case-by-case basis, but in the first place, “I am trying to guess something that is quite difficult to guess” or that the data collection method is not appropriate .
The second is related to that, but it is "I made it, but I can't get it to be used by the people I was expecting, people in the company, or customers." If you can not use it, if it is a problem with accuracy, you need to improve accuracy, and there may be problems with UI and UX.
We also think that lowering the expected value is an effective approach. For example, a Chatbot that supports customers requires a high degree of accuracy, but I think Microsoft's high school girl AI Rinna is a good example of lowering expectations. It's a design that is acceptable even if it says something strange. I think it's an effective approach to start from a place where the expected value is low or a certain degree of error is allowed in the business process .
--Isn't lowering the expectation sounding backwards?
Takeda : No, I think it would be better if we could continue to work on this and improve the accuracy. The accuracy that we initially aimed for does not come up suddenly, so it is often successful to reduce the expected value as much as possible and collect evaluation data, aiming for accuracy that gradually produces a business impact.
Takeda : The third story I often hear is that I bought a service that has an external AI, but I can't use it as I want. That is also one of the problems in the introduction of AI.
Examples of failures that are not shared with value
-It seems difficult to judge how much accuracy you can achieve for what you want to achieve, but is it understandable?
Takeda : Of course, it is difficult to guarantee accuracy, but if you look at an engineer who has made a model, you can get an idea. I think that it is empirically clear that it is impossible to do it or if you try hard enough.
As one of the judgment criteria, I think it is quite difficult for people that cannot be judged. Even in the cases of large companies in the past, there were some cases where it did not work well with the goal of making things that humans could not judge. It is natural for me to make mistakes, but I don't quite say it out. A very large failure may be heard.
Shimamoto : I participated in an accelerator program specialized in AI this time, and it was very fresh and inspired. Many AI startups are starting out as consignment in the early days, so it seems that they have failed everywhere, but it seems that they have not appeared online. Since it may be shared in a small community, I felt that the information about the failure was important.
* The dialogue will continue to the second half. Click here for the second half of the article
---
For those who want to consult with DataStrategy
DataStrategy accepts consultations such as writing, event presentation, coverage, service improvement, advisory, etc. If you would like to talk to us first, or if you would like to hear the story, please DM us on twitter ( @motohikotakeda ).
・AI possibility diagnosis https://datastrategy.jp/aikanousei/
・Our website https://datastrategy.jp
・Document request https://datastrategy.jp/document/
・Our website https://datastrategy.jp
・Document request https://datastrategy.jp/document/
Reprinted for study.
Motohiko Takeda / CEO, DataStrategy Inc.
Founded DataStrategy, who is a freelance data scientist and directs the overall technology introduction for new business development, business automation, and data-driven marketing. Teikyo University Lecturer (Marketing Science), Knowledge Merchant Works Co., Ltd. Outside Director (Data Science)
*I 'm talking with Mr. Shimamoto, the representative of AnyTech , about the reasons why AI isn't working well . This article will come later. Part of the article is here from
The most important thing is how to plan properly. Consider replanning even if it's not working
--This time, I would like to talk about your own experience of "if you do this, it worked" and "it works."
Takeda : I think planning in advance is the most important. With such data and technology, it is important to plan in advance that it will be possible to achieve such accuracy and to verify it, and develop what can be actually used.
If task setting is difficult, accuracy will not come out, and if accuracy does come out and there is no impact on the business (P/L), when I look back later I might be wondering what was happening? not. It is necessary to set the task from the perspective of whether the accuracy is likely to occur or the business impact is likely.
The ways in which the impact of AI introduction on the business can be broadly classified into those for customers (incorporating into products) and those for internal use (cost reduction). For customers, the level of accuracy required for AI will also increase, but for internal use, using AI in combination with in-house personnel will improve the accuracy of work as a result, rather than using AI alone. There are times when you want to increase or increase efficiency.
Based on existing data and technology, it is necessary to properly design what level is likely to be achieved and how to incorporate it into the business.
Shimamoto : I ended up talking about planning. I think that it is important to consider it as planning that incorporates solid verification and development of a Proof of Concept (Procedure for Proof of Concept) into planning.
It is common to recognize that the conventional planning is just before the development is undertaken, and then carefully plan the plan before starting. However, in the case of AI development, it is possible to plan with a certain degree of accuracy only by creating a PoC, so I think it is important to proceed with the project on the assumption that there is a PoC in the planning . If you don't do that, the initial planning will be an empty theory on the desk, and the budget, the period, and the accuracy will be misaligned as a whole.
--For example, what should you do if you have already introduced AI but the accuracy does not improve?
Takeda : There are 3 steps. Reproducing the decisions made by humans is the fastest way to improve accuracy, so the first step is to understand exactly what the person in charge of the job sees when making decisions. With that in mind, the second is "let's properly convert the information that the person uses to make decisions into data". The third is "Let's do our best by improving the algorithm".
You may generally think that it would be more accurate if you brought someone who could develop a great algorithm. Of course that is also important. However, if the data is useless, it will be difficult for the algorithm to do its best, so the royal road is to make the data rich and try the algorithm.
Shimamoto : I also have two things, and I have to re-examine and revise the tuning method. Tuning is quite human-powered, or has a lot of belongings (belonging to that person), and there is no correct answer that this method is good for this business, so there is room for review. There may be a way to consult with a company that has a different method.
On the other hand, there are many cases where the second problem is not a tuning problem, so it is quite painful as a business decision to review from the first design, or it is painful as a loss cut, but that is smoother. There are also patterns. On the other hand, it may be similar to conventional system development. Although I made it halfway, it means that the design was not good in the first place.
The importance of choosing an AI development partner
--I think that you often need a partner to proceed with AI development. Can you tell us about choosing a partner for AI development?
Takeda : AI technology is extremely widespread and is evolving day by day. Human resources who can analyze data related to AI are also very popular, and given the tight supply and demand situation, it is unrealistic to provide the knowledge and human resources necessary for introducing AI within a single company. I think it is.
I think it's necessary to choose the right partner at the right time and to "use it" in a good sense, without trying to force everything in-house.
I also often hear that "I don't have access to good ventures." I don't know where the technology is in the first place. Even if it doesn't happen, I think it's important to access a good community on a regular basis. I think that around the ventures with technology, there are ventures with technology properly, and around the engineers with technology, there are engineers with technology.
Shimamoto : AnyTech is a community of top engineers who work for freelance and non-professional jobs. From that experience, in addition to the story that each expert is needed, there are two more.
One is whether or not he has failed in that area. Where there is a high level of technical skill, there is a wealth of experience with failures, so that is one sign (decision factor).
The other is that failure alone is not a problem, and whether it is an iterative cycle (development cycle in software development, especially in agile development that is repeated in short intervals). I think it will be a good indicator of a partner whether it takes a year or two to achieve one milestone, and whether or not it can be subdivided and iterated. Since new technologies are coming out sooner, whether or not they can be adopted is also the speed of iteration and is important.
AnyTech is also a development service group that has accumulated experience of failures through various developments and can understand the point of setting the appropriate cycle.
---
For those who want to consult with DataStrategy
DataStrategy accepts consultations such as writing, event presentation, coverage, service improvement, advisory, etc. If you would like to talk to us first, or if you would like to hear the story, please DM us on twitter ( @motohikotakeda ).
・AI possibility diagnosis https://datastrategy.jp/aikanousei/
・Our website https://datastrategy.jp
・Document request https://datastrategy.jp/document/
・Our website https://datastrategy.jp
・Document request https://datastrategy.jp/document/
0 コメント:
コメントを投稿